LeAK & AnGer
Automatic Anonymisation and Pseudonymisation of Court Decisions
German courts are legally required to publish their verdict, but according to estimates, only a small amount (under 3%) of all court decisions are published per year. The transparency and availability of such documents do not only grant legal professionals and the general public access to crucial information source, but is also of importance for legal-tech industry and the digitalisation of government institutions. The main reason for this is the need of a time-consuming and yet manually anonymisation process. Also, to the best of our knowledge there is no high-quality German corpus available to train a fully automatic model and most existing tools are still semi-automatic systems. Hence, they only provide a support for the manually anonymisation process. In LeAK and AnGer, we want to fill these gaps by working on the creation of high-quality annotated training data using verdicts from different law domains and developing a prototype for a fully automatic anonymisation.
AnGer
- Start date: 01.01.2023
- End date: 31.12.2025
- Funding source: Bundesministerium für Billdung und Forschung (BMBF)
Motivated by the promising results from our prior works in LeAK, the main objectives of the AnGer project lie in the further development of our automatic anonymisation system and the creation of a new high-quality annotated dataset consisting of court decisions from higher regional court (Oberlandesgericht). Moreover, the models generalisation across different law domains is still a challenging task which should be addressed in this project. We are working on different data augmentation techniques, as well as robust neural networks which help to enhance the robustness of our current prototype. Especially, data augmentation can also help to generate more training samples for domains that lack of training data. Further, according to our findings in LeAK, domain adaptation is still required in certain domains. Thus, we create learning curves for AG and OLG datasets to visualise the learning quality during each training step and to analyse the amount of data needed for a robust domain adaption. We also work on an approach for continuous domain adaptation without retraining using all data. Finally, we want to carry out legal-tech studies and conduct de-anonymisation experiments with the annotated gold standard.
LeaK
- Start date: 01.04.2020
- End date: 31.03.2022
- Funding source: Bayerisches Staatsministerium der Justiz (StMJ)
This project aims at exploring the feasibility of a fully automatic anonymisation system for German court decision. One of our key contributions is the development of a high-quality manually annotated gold standard for verdicts from regional districts (Amtsgericht). To ensure the absence of privacy-related information, at least six people have to work on the same document. Therefore, each text in our dataset is annotated independently by four student annotators, who have to identify text spans that need to be anonymised, information categories (names, addresses, jobs or dates) as well as risk levels (high, middle and low) and adjudicated by two new annotators in the subsequent step. Text anonymisation is thus approached as Named Entity Recognition (NER). Another essential aspect of the project is the systematic evaluation of different automatic approaches for automatic anonymisation using existing NER taggers, as well as fine-tuning several Large Language Models (LLMs) on our gold standard. Especially, we are also working on a multitask architecture in order to enhance the robustness of the anonymisation system. In the course of these experiments, the transferability of our prototype should also be validated across documents from other law domains of higher regional court (Oberlandesgericht) . Preliminary results of this experiment indicate the need of domain adaption in order to achieve good performance on court decisions from other text domains.
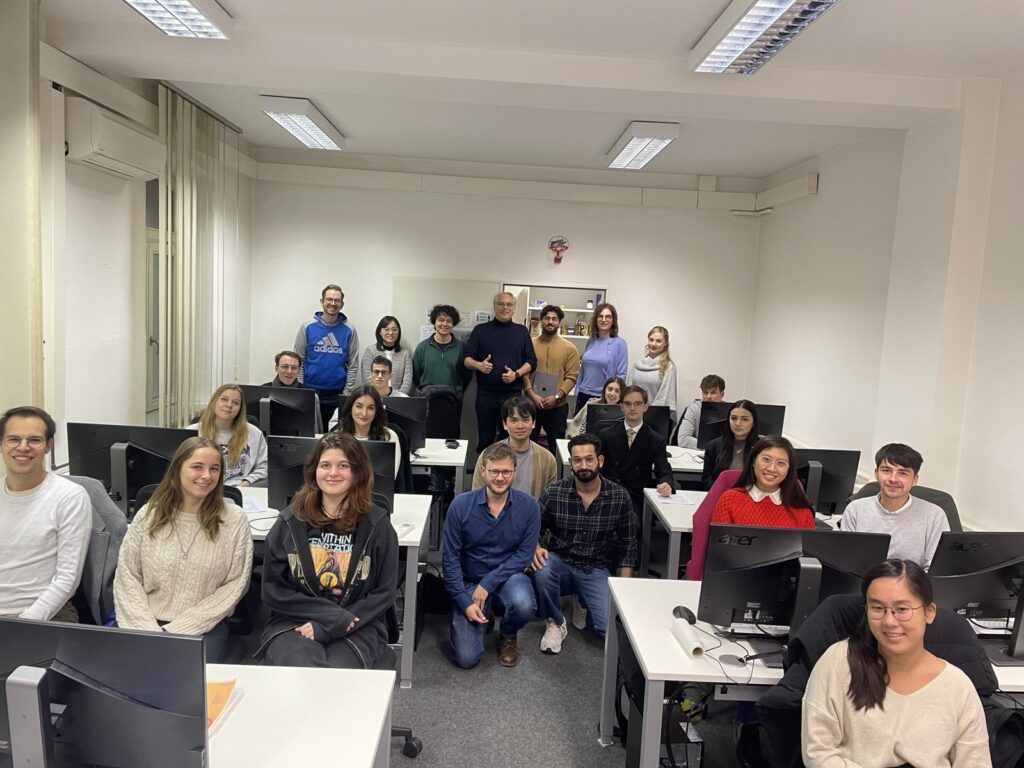