Results
Initial results with Amtsgericht (AG) data
We fine-tuned GottBERT, a German pretrained language model, using this corpus and got a F1=84% for all anonymised data points on our test set which indicates great potential for anonymisation. Especially, we could reach a Recall of 96% for PII (personal identifiable information) text spans
Text spans |
Recall according to risk | |||||
Precision | Recall | F1 | High |
Medium |
Low |
|
GottBERT | 0.80 | 0.90 | 0.84 | 0.96 | 0.80 | 0.89 |
In order to get more robustness, we also built a joint learning model which is trained to solve three different tasks simultaneously using the same corpus, namely, spans detection, entity classification and risk prediction. We achieved a Recall of around 98.8% for PII text spans and a total F1=96.56% (Precision=96.88, Recall=96.24) across all AG domains.
Current cross-domain results with Oberlandesgericht (OLG) data
To evaluate the generalisability of the multitask model, we ran additional evaluations using verdicts from 10 law domains from higher regional courts (Oberlandesgericht). Recall values for PII text spans show that our multitask model (trained with AG data only) could already detect most of the high risk text spans for all OLG domains except for Immaterialgüter. Additionally, we developed an AG+OLG combined model by using both datasets and received substantial improvements in all OLG domains compared to initial cross-domain validation. The table below also shows numbers of training tokens, documents within each law domain in our OLG training samples.
OLG domains | Precision | Recall | Recall (PII) | Recall AG+OLG | Recall AG+OLG (PII) | n Training Tokens (OLG) |
n Training Documents (OLG) |
n Tokens/Document (OLG) |
Allg. Zivilsachen | 89.22 | 93.61 | 97.91 | 96.26 | 99.30 | 112300 | 42 | 2673,80 |
Bankensachen | 92.89 | 93.83 | 100.0 | 96.51 | 100.00 | 49530 | 26 | 1905 |
Bausachen | 94.48 | 97.13 | 94.86 | 98.16 | 96.37 | 59715 | 18 | 3317,5 |
Beschwerdeverfahren | 80.58 | 95.91 | 93.83 | 95.57 | 98.77 | 42872 | 18 | 2381,77 |
Familiensachen | 86.15 | 92.63 | 95.40 | 94.02 | 98.28 | 37691 | 17 | 2217,11 |
Handelssachen | 84.90 | 95.08 | 99.07 | 97.63 | 100.00 | 109228 | 24 | 4551,16 |
Immaterialgüter | 78.65 | 77.36 | 83.90 | 83.11 | 87.80 | 87367 | 21 | 4160,33 |
Kostensachen | 85.53 | 94.67 | 100.0 | 98.00 | 100.00 | 11028 | 8 | 1378,5 |
Schiedssachen | 90.24 | 85.84 | 97.87 | 94.56 | 98.94 | 35399 | 12 | 2949,91 |
Verkehrsunfallsachen | 86.02 | 85.35 | 95.88 | 89.90 | 98.24 | 63300 | 24 | 2637,5 |
Learning curves
The graph below describes the anonymisation performance with different data sizes and suggests some room for improvements, as the curves continue to rise steadily, especially finetuning an OLG model still requires more data since the best Recall is just around 93% for all LLMs we used during the experiments. This once again illustrates the importance of domain adaptation.
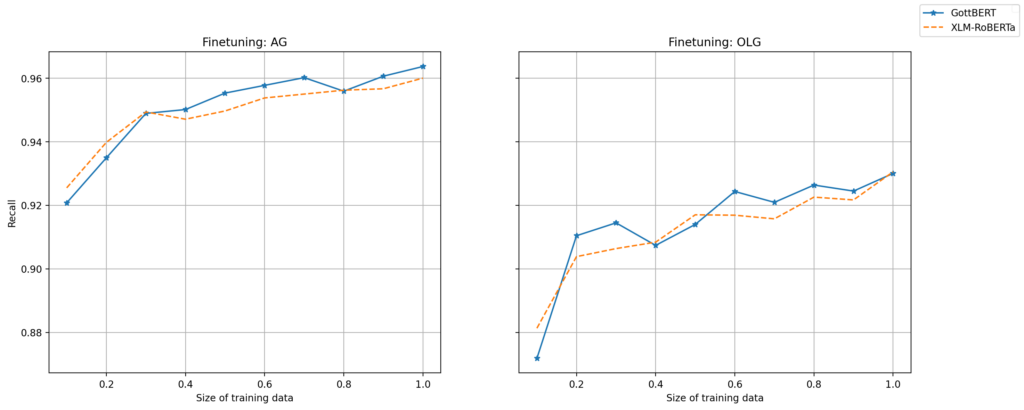
For further details about our experiments, please consider taking a look at the following blog posts: